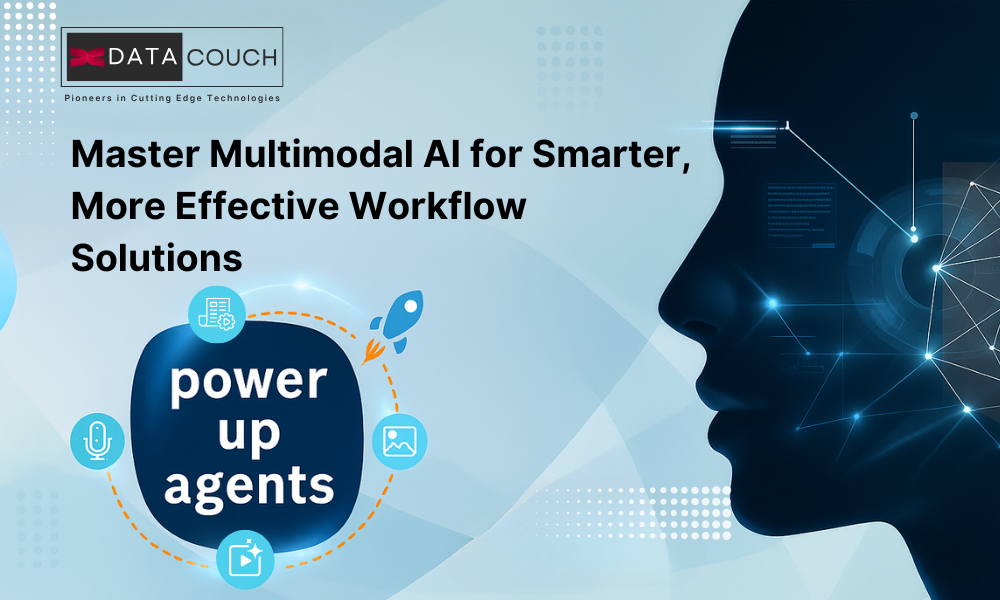
When to use Multimodal Agents?
Understanding the Role of Multimodal AI in Modern Agents and Choosing the Right Multimodal Model for Your AI Workflow
Introduction
Imagine asking your virtual assistant to not only answer your questions but also analyze an image, translate a sign, and suggest personalized insights—this is the power of multimodal agents. As AI evolves, knowing when to leverage these agents becomes critical.
If you’ve been keeping up with the latest AI model releases, you’ll notice a common trend in every major launch–every new release comes with better Multimodal capabilities. Whether it’s Mistral 3.1, Gemma 3, Gemini 2.5, Claude 3.7 Sonnet, or ChatGPT 4o, each LLM flaunts its better, efficient, and more precise multimodal capabilities.
Even when it comes to combining multiple Agents, models, and tools to solve a complex task, this feature has become a must-have. But, understanding when and where to use it is crucial. A clear understanding of real-world use cases of multimodal AI agents help you to combine agents with multimodal models to enhance contextual responses.
In this article, we’ll break down how Agents integrate with Multimodal AI and guide you in choosing the right open-source or proprietary model for your needs.
Learning Objectives
- Understand the meaning and significance of Multimodal AI and Agents.
- Identify real-world problems where Multimodal Agents excel.
- Learn how to choose the best multimodal AI model – open-source or proprietary.
- Discover why Google’s Gemini and Gemma models stand out in multimodal processing.
What is Multimodal?
In the AI domain, we have seen three major waves: Predictive Maintenance, Generative Models, and AI Agents. A common challenge across all of them is obtaining and preprocessing data. While autoregressive models have made text-based data widely accessible, handling images, video, and audio remains complex. Traditional AI systems typically process only one form of data, usually text. However, real-world information is diverse and often requires a combination of inputs to derive meaningful insights.
This is where the concept of “Multimodal” AI becomes crucial. In simple words, Multimodal AI refers to the integration of different types of data, such as images, videos, audio, and text into a single, unified model. Multimodal AI enables models to analyze multiple data formats simultaneously, leading to richer and more context-aware responses. For example, in customer support, a Multimodal Agent can process both text inquiries and attached images to provide more accurate and relevant assistance.
Multimodal Agents: All You Need
Now that you understand what Multimodal is in words, let’s understand how this can be related to AI Agents.
What are AI Agents?
An Agent is an autonomous system designed to interact with its environment by using Ai models, tools, memory, and planning mechanisms. It processes inputs, decides a plan of action, executes tasks, and continuously refines its approach through self-reflection and self-criticism.
Furthermore, an Agent can retain contextual information with short-term and long-term memory to recall past interactions to enhance decision-making. Additionally, it can employ structured reasoning techniques such as subgoal decomposition and iterative feedback loops to optimize task execution. Typically, the interaction with human users or supervisors is through text-based input, prompts, responses, and feedback.
What would Multimodal AI Agents do?
When extended to Multimodal Agents, the capability expands beyond text-based interactions to incorporate multiple data formats, including images, audio, and video. Unlike traditional Agents that rely solely on textual input, Multimodal Agents process and integrate diverse data sources to generate more comprehensive and context-aware responses. Let me give you one example that defines a very good use case for the Multimodal Agents.
Have you ever been to a shopping mall, checked the ingredients list on a product, and then searched for those keywords to understand their nutritional value? With a Multimodal LLM like GPT, Claude, or Gemini, you can simply click and upload the ingredients list as input. Then, they can analyze and describe the impact of each of the ingredients on you in plain English (or any other language of your choice). Now, imagine if you could combine this capability with tools like Google search, a specialized nutritional API, and an allergy detection model. The agent not only fetches detailed ingredient information but also checks the ingredients against known allergens and provides personalized dietary recommendations. Combining this with contextual memory, the Agent can retain information about your dietary preferences, allergens, and health goals to offer highly accurate and tailored insights.
A Business Use Case for Multimodal AI Agents
Managers often deal with multiple streams of data—video recordings of meetings, past customer interactions, feedback reports, and sales performance metrics. Manually analyzing this data to make informed decisions can be time-consuming and prone to errors, especially when it comes to onboarding new associates and team members.
For example, an executive can upload a recent video recording of a customer focus group to understand feedback on a new product. The agent can transcribe and summarize the key points from the video, cross-reference this information with past customer feedback, and analyze sentiment patterns. Additionally, it can compare this data with sales trends to highlight correlations or discrepancies.
Based on this comprehensive analysis, the agent generates a detailed report outlining different customer scenarios—positive, neutral, and negative—and suggests appropriate responses for each. It may also recommend changes in marketing strategy or product improvements to better align with customer preferences.
Real-time Applications of Multimodal Agents
In the Retail space, Multimodal AI systems analyze customer inputs in real time to deliver personalized recommendations. For example, a shopper might upload an image of a product or style they like, and the system instantly cross-references this with textual reviews, browsing history, and even social media trends. By using multimodal agents for retail personalization, e-commerce platforms can suggest complementary items or similar products in seconds, enhancing the shopping experience and boosting conversion rates.
In Agriculture, multimodal AI for agriculture and farm management are transforming farm management. Drones capture live images of crop in fields while sensors continuously monitor soil moisture, and weather stations feed in climate data. By merging these diverse data streams on the fly, the system can detect early signs of pest infestations or nutrient deficiencies, allowing farmers to intervene immediately. This timely decision-making helps optimize resource usage and improve crop yields while reducing costs.
Customer service operations also benefit from real-time multimodal AI. A multimodal AI for customer service automation can simultaneously process voice calls, chat logs, and video interactions to gauge customer sentiment. This real-time analysis enables the system to identify urgent issues, automatically prioritize tickets, and provide tailored responses based on a holistic view of the interaction. The result is faster issue resolution, higher customer satisfaction, and a more efficient support process overall.
What Multimodal model should you use?
Recent evaluations reveal notable differences in the multimodal capabilities of leading Large Language Models. OpenAI’s GPT-4o performs exceptionally well in conversational image editing and Visual Question Answering(VQAs) but falls short in object detection when compared to domain-specific models. Claude 3.7 Sonnet shows strength in text-to-speech synthesis and multilingual understanding, yet it trails behind Google’s Gemini 2.0 Flash in complex Multimodal reasoning benchmarks like MMMU.
Interestingly, Gemini 2.0 Flash despite not a dedicated reasoning model that delivers strong results on Multimodal tasks. Its successor, Gemini 2.5 Pro (experimental), currently holds the highest vision ELO score at 1315, further establishing Google’s edge in this domain.
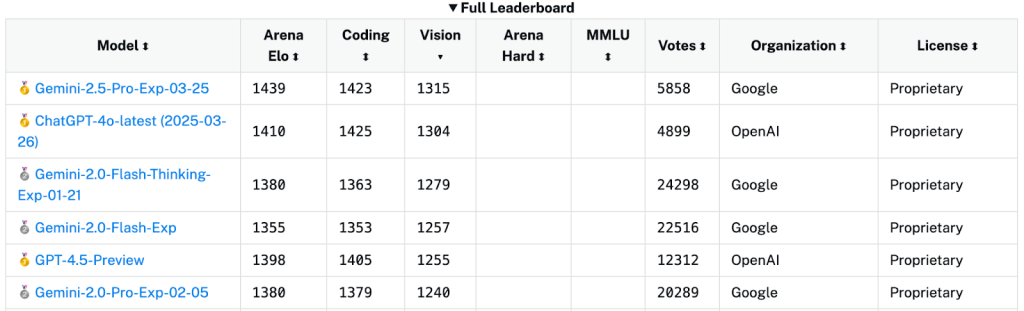
Google’s Gemini 2.0 Flash, with its 1 M-token context window and native tool usage, leads benchmarks like MMMU and LiveCodeBench, surpassing models such as GPT-4o and Claude 3.7 Sonnet in multimodal reasoning. On the open-source front among all the open-source multimodal AI models for developers, Gemma-3 27B strikes a strong balance between efficiency and scalability. It scores 1341 on the Chatbot Arena ELO ranking and outperforms LLaMA 3 405B and DeepSeek V3 in various multimodal tasks. It also supports fine-tuning for specific use cases, including object detection.
For vision-focused multimodal applications, the Gemini series is a solid choice, while Gemma-3 27B offers a powerful open-source alternative with customization flexibility.
Conclusion
As industries increasingly rely on real-time, data-driven insights, adopting Multimodal AI becomes beneficial and essential. In this blog, we’ve explored what Multimodal means, how Multimodal Agents work, their real-time applications, and the factors to consider when choosing the right Multimodal AI model. We’ve also explored the benefits of multimodal AI agents in enterprise settings including the comparison of available enterprise multimodal models. In the next article, we will focus on how to build a multimodal AI agent with code that is powered by real-time search capabilities.